Admitted PhD students. If you have already been admitted and are looking for potential advisors, please email me directly.
Prospective postdocs. Please email me with your CV, your research statement, and a description of your future research plans.
UW PhD students. I'm generally happy to collaborate -- send me a message on Slack!
UW undergrad or MS students. I do not currently have research openings for specific ongoing projects. If you're interested in working with us, I'm teaching a ML Capstone in Spring 2025 that will have a significant course research project component; please consider enrolling for that. If you have prior research experience and a specific project/direction that you'd like to collaborate on, please feel free to email me.
Students outside of UW. Sorry, I am generally not taking any visiting students from outside of UW. If you have a specific project in mind that you would like to collaborate on, please feel free to email me.
What will you be like as an advisor?
My goal as an advisor is to help my students develop into independent researchers who are driving their own impactful research agendas. My role is to support your growth and enable you to do your best work. This support takes various forms, both technical (e.g., identifying high-level research directions, and working through on low-level problems together) and non-technical (e.g., discussing career opportunities and trajectories, and making sure you have a supportive work environment). Ultimately, the purpose of the PhD program is to develop students. My aim is for you to finish your PhD not just with several impactful papers to your name, but to be ready to go forth and become a leader in the research community in your own right.
What does this mean concretely? First, you should feel empowered to take ownership of your research direction. I’m broadly interested in many topics in AI, and I aim to be hands-on in terms of working together to define and execute on problems, but flexible in terms of research direction and giving you the space to develop your own ideas. Of course, we’ll still work closely together – especially at the start – to brainstorm ideas that we’re both excited about and refine them. But, I expect you to learn to be independent and to take the initiative to drive your own research direction.
Second, it means that we will work towards your long-term success, even if it can sometimes be at odds with short-term progress. As examples: coming up with your own research ideas can be slower at the start, but it’s a critical skill to hone; when we select projects, we will balance exploitation with exploration of new areas and skills that you’d like to grow in; when we’re writing up a paper, I’ll give several rounds of feedback instead of directly editing it; and we will take our time to work on solid, high-impact projects instead of always optimizing for the next deadline.
What kind of research do you want to do?
I am broadly interested in making machine learning models more useful in real-world applications. What are the ways in which they are currently not meeting our needs, and how can we develop principled ways of understanding these failure modes and mitigating them? It’s a really exciting time for machine learning research, and I want to build the scientific foundations for how we can harness all of the new developments in machine learning and bring them to bear in societally important applications such as biomedicine, public health, scientific discovery, conservation, and education.
To get a more concrete sense of what I work on, check out my webpage and recent publications. But that is not an exhaustive list of all the topics I might be interested in. I’d be very happy to work together to brainstorm on common interests and help you carve out your own direction that you’re excited about! And you are very much encouraged to pitch me on what you’re excited about.
In terms of style, I value research that is simple, clean, scientifically rigorous, and conceptually interesting. I want to deeply understand what we’re doing and why it works (or not), through the lens of theory and/or experiments. I strive to identify problems that are underexplored and relevant to real-world applications. Many of my favorite papers are about defining a problem in the right way and then providing a simple solution to it. And where possible, we should keep ourselves honest and make sure that our methods actually work in the real world.
What about collaboration and co-advising?
I firmly believe in the benefits of collaboration. As a personal example, all but one of my first-authored papers during my PhD were co-first-authored, and being able to work with great coauthors and collaborators made my PhD much happier and more productive. I encourage a similarly collaborative culture in my lab, and my goal is to make it easy for students to work with others within and outside of the lab. But collaboration is of course not mandatory, and it’s a good learning experience to have at least some work that’s done alone.
Collaborations can come in many forms: working with a peer together on a problem; mentoring a junior student or being mentored by a more senior student; or working with an expert from a different discipline. Each type of collaboration has its own rewards and challenges, but proactive communication and coordination can resolve many potential issues, and the ability to collaborate effectively is a very useful skill to develop.
Practically, this means that you should feel free to explore collaborations with other labmates and with students and faculty outside the lab (or even department or school). I would also be very happy to explore co-advising, if and when you want to, but co-advising is definitely not a prerequisite to being able to collaborate with others. I’ve found UW to be a uniquely collaborative environment where students and faculty from different groups often mingle and work together.
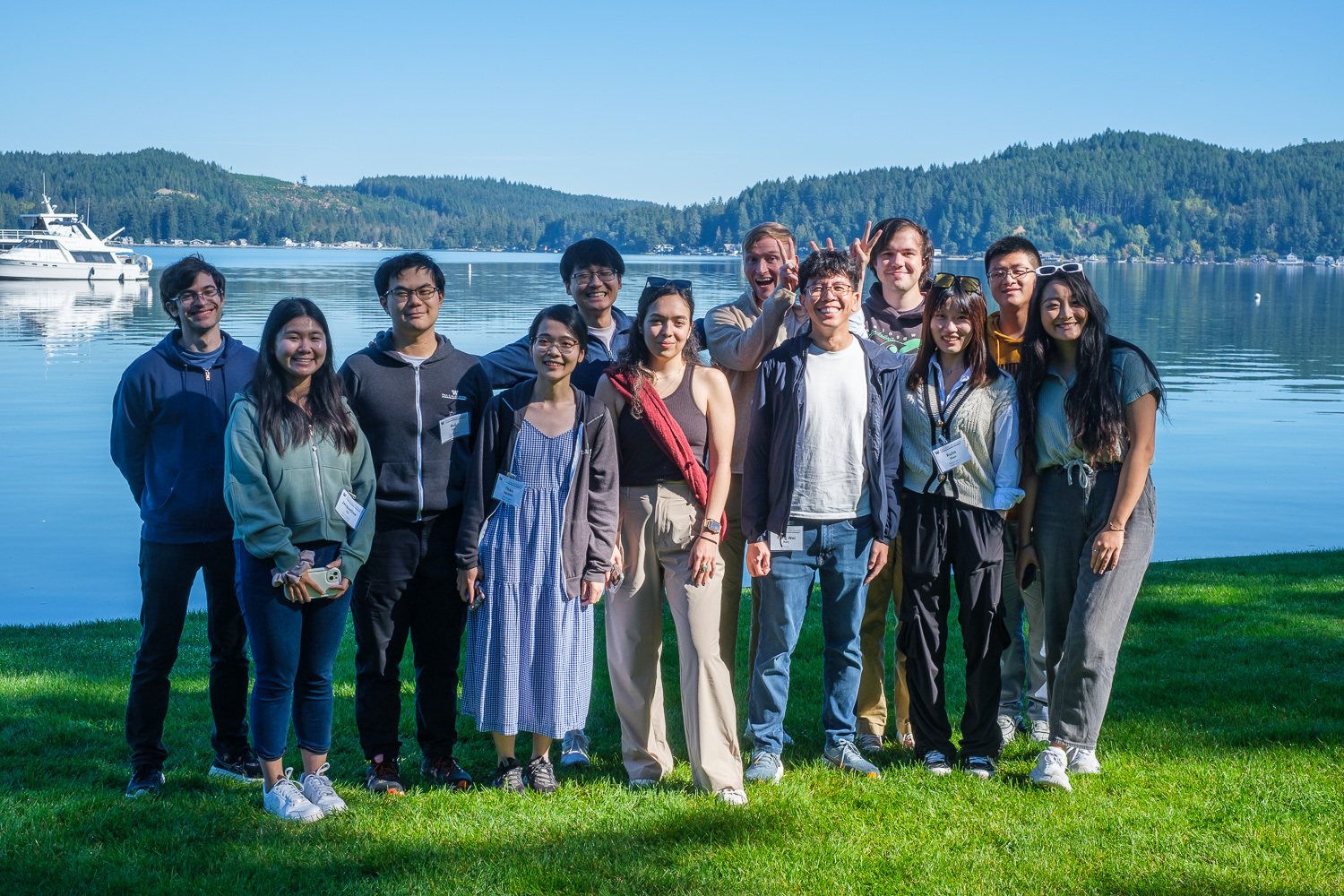